Learning from Covid: 4 data lessons
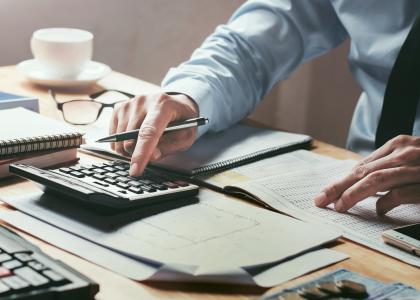
To respond to a crisis, we’re told that decision makers need to look at the data and “follow the science”. While this may seem desirable in theory, recent events demonstrate that it’s sometimes far from easy in practice.
From the R-rate right through to a rolling stream of behavioural indicators, headlines over the last few months have been dominated by data. Here’s how the management, interpretation and presentation of this information by policymakers highlights wider lessons that all organisations can learn from.
1. Data rarely arrives neatly bundled
When a previously encountered situation arises (seasonal flu or a temporary supply chain break, for instance), we have the benefit of hindsight. We can take the data linked to what happened last time around and use this as a base model for predicting what’s likely to happen next.
With a novel situation, there is no useful base model to refer to. With Covid-19, analysts were starting from scratch, with little or no knowledge about spread patterns, viral evolution, cultural differences and other key medical and demographic factors. The learning curve has been steep.
Policymakers have been required to manage and make sense of an ongoing deluge of information. This covers everything from statistics recorded by clinical facilities on the ground, right through to academic research findings and information from government bodies from across the globe on the effectiveness of the actions they’ve taken.
Before this data can be interpreted, it firstly has to be processed; something that’s not always easy. Much of it is unstructured, with the possibility that key insights are buried deep in the narrative. There will be contradictions between different data sources, and sometimes internal inconsistencies within the same dataset. Integrity is a further issue: after all, some data sources will always be more reliable than others.
The lesson
- With data, there is always the temptation to focus solely on the ‘low-hanging fruit’. For instance, if you take the homegrown data derived from your internal systems as your sole basis for analysis. After all, you’re familiar with it, you can vouch for the way it has been collated, and it will be easy to integrate it into a model for analysis.
- But if you focus on data from a very select group of internal sources, it can severely limit the value and usefulness of your analytics project. It also increases the risk of bias: integrating data from certain sources and ruling out others, simply because other sources are too difficult to integrate, or because the content is likely to result in unwelcome conclusions.
- Just like policymakers, organisations need the full picture. This includes the ability to explore and analyse structured, unstructured, internal, external and public content. The end goal should be to uncover trends and patterns that improve decision making: even if those insights are contained within ‘difficult’ sources.
2. Presenting your data story
The UK government’s daily televised Covid-19 briefings have recently been brought to a close. If you were a regular viewer, you’ll know that slideshows covering matters such as infection rates, hospital admissions and mobility patterns featured heavily. The idea was to explain the story of the country’s Covid response through data.
You may also have noticed a significant change in how that information was presented. Early on, the UK’s data covering metrics such as infection rates and death rates were shown side-by-side with a host of other countries. After a few weeks, they stopped doing this as often.
Critics said this had happened purely because the UK’s trajectory compared unfavourably with many other countries. Officials had a different explanation. They pointed to the fact that diagnosis and reporting procedures can differ widely between nation states. Where different protocols are being followed, like-for-like comparison can be both unhelpful and misleading.
The lesson
- Organisations - and even companies within a single group - can develop their own ‘data cultures’, with different priorities and processing methods. It can be particularly noticeable where two businesses merge, each with its own preferred data methodologies.
- Without the right governance in place, there’s a danger of different business units becoming data islands. Fragmented, incompatible data makes it difficult to get an organisation-wide view of performance.
- Centralising data sources across your organisation can ensure that each business division has access to a common source of trusted data. It helps to ensure that you are working to consistent benchmarks, comparing like-for-like and telling a consistent data story. All of this can mean more confident decision making.
3. From anecdote to evidence
In the early stages of the pandemic, the NHS listed two recognised symptoms in its official guidance: a persistent cough, and fever.
But at the same time, a steady stream of reports were coming through of patients reporting anosmia (loss of taste and smell). Before considering including this on the list of symptoms, policymakers needed evidence on both the prevalence of anosmia among Covid sufferers, and its usefulness as a predictor of the disease.
Concrete evidence came in the form of a joint analysis involving Massachusetts General Hospital, King’s College London, the University of Nottingham and health science company, ZOE. Analysing the data gathered from 2.5 million people in the US and UK, researchers were able to establish a very clear link between anosmia and onset of the disease. They also created a mathematical model capable of predicting with nearly 80% accuracy the likelihood of an individual having the illness, based on their characteristics and symptoms.
The lesson
- Data analytics is often touted as a way of avoiding making decisions on a hunch. This is true, but it can also be used to test assumptions and investigate anecdotal experiences.
- For instance, let’s say you receive reports from your customer service team that a certain product line is ‘more trouble than it is worth’. Topline sales figures appear strong. And yet, dig a little deeper, you discover that resources expended on aftercare and queries significantly impact this product line’s true profitability.
- Often, a ‘hunch’ can be right. Data analytics provides the evidence needed both to back it up, and to formulate the right response.
4. Timely decision making is a brand reputational issue
When the chancellor, Rishi Sunak opened up his initial government-backed loan scheme, an estimated 300,000 businesses rushed to apply. And yet, three weeks later, it was reported that only 1.4% of businesses that had enquired about the scheme had been successful in securing funding.
In the current landscape especially, businesses in need of credit or borrowing arrangements need quick decisions. At the same time, financial institutions have their own priorities to juggle. Many are expected to do more with less; to speed up application processing, while reducing manual input. There’s also the need to ensure that institutional lending criteria are met, and to manage lending portfolio risk.
As PwC points out in its assessment of post-Covid banking practice, “How you respond to your customers could be pivotal to how your brand is seen for years to come”. Empathy is key to this - and so is the ability to respond quickly to customer needs.
The lesson
- The ability to make timely, evidence-based decisions is often characterised as an operational This is correct, but it can be crucial for reputational reasons, too.
- This can be especially relevant in the analysis of credit risk, including setting criteria for extending credit lines and granting payment relief.
- To determine the type of arrangements you are able to offer customers, you need the ability to assess organisational drivers, react to unpredictable conditions and weigh up your options.
Future-proofing your data analytics capabilities
In our recent webinar, we explored how IBM Planning Analytics can give organisations the speed and agility to respond to even the most complex and volatile conditions.
To bring multiple data sources together, to plan, forecast, test assumptions and make adjustments, discover how IBM Planning Analytics can help you make the right decisions.