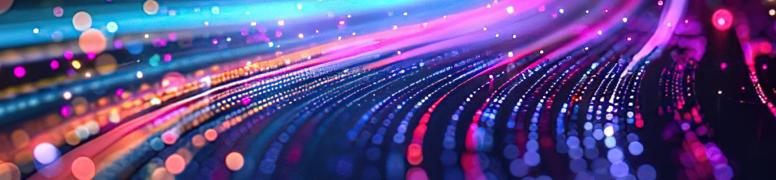
ChatGPT – How does ChatGPT deal with toxicity?
02/05/2023
One of the major problems OpenAI had to overcome in order to realise ChatGPT, was one inherited from the GPT-3 model, in that it was susceptible to coming out with violent, sexist and racist content.
This was because it was trained on publicly available sources of data like the internet, which inherently contains a lot of toxicity and bias. Simply manually sifting through such masses of data by hundreds of employees would take decades to complete.
OpenAI took a leaf out of Meta's book and built an AI toxicity detector that recognized such text to a) prevent any such text being relayed back to a user in the context of conversation and b) help filter such text from being put forward towards the model in the first place.
Meta had previously built a similar tool to detect toxic posts. This would involve building a model from labelled examples including but not limited to violence, hate speech, and sexual abuse.
Unfortunately to get those labels, OpenAI outsourced this work to a company called Sama, a San Francisco based outsourcing firm, that employs workers in Kenya, Uganda and India to label data for Silicon Valley clients like Google, Meta and Microsoft.
These workers are paid a very low wage of between $1.32 and $2 per hour to label data. However, it's the mental scars that are the worst to bear with having to deal with such content daily.
Stories like this are not normally as widely known as the success stories of the tech itself. But we think it’s important for people to know the sacrifices others are making for us to benefit from safe technology.
We asked ChatGPT our question and this is the response:
ChatGPT, like other AI models, is trained on large amounts of text data from the internet. This data can include examples of toxic or offensive language. As a result, the model may generate responses that are also toxic or offensive. To mitigate this, OpenAI has implemented a number of filters and mechanisms to detect and remove potentially harmful content generated by the model. Additionally, the team is continuously working on improving the model's ability to generate safe and appropriate responses, by fine-tuning the model on more diverse and less biased data. However, it's worth noting that the model is not perfect and it may generate inappropriate responses.